Tung-Hui Hu is an Associate Professor of English at the University of Michigan, a published poet, and a former network engineer. His first book, A Prehistory of the Cloud published in 2016, illustrates how the cloud grew out of older digital networks, and “examines the gap between the real and the virtual in our understanding of the cloud.” (Hu, 2016)
In his most recent published work, Digital Lethargy, Hu examines the phenomena of exhaustion and lethargy shared by digital users in an age of digital capitalism, where concepts of ‘liveliness’ and ‘agency’ are sold to users for the benefit of big tech and advertisers, specifically with data. Digital platforms created by tech industrialists gather data from “active users” through their clicks, likes, status updates, and even their racial and gender identity in order to generate profits from advertisers.
“Under digital capitalism, ‘being yourself’ is the dominant set of codes for how we understand ourselves and others. It is a form of empowered individualism, where we equate a user account with personhood, and we equate choice with agency.” (Hu, 2022, viii)
This results in users carrying the heavy burden of ‘performative livelihood’, in which personhood is intrinsically linked to the digital self, leaving little to no room for identity outside of digital capitalism. Instead of shaming feelings or acts of lethargy, Hu argues that lethargy in a digital capitalist system can be a form of resistance itself. It can be a response to a disconnect; in a digital system where communications are tracked, collected, and analyzed as data, the lack of relation to others can indicate participants refusal to adhere to scripted and algorithmic modes of “connectedness”.
Hu makes the point that privileged concepts of ‘agency’ and ‘choice’ presented by digital platforms are “not afforded to all populations in the same ways.” (Hu, 2022, xiv) In digital capitalism, microwork is typically employed to those who can afford to work long, menial tasks with little pay, given the circumstances they were born into.
“‘Robotic’ work takes place in countries like the Philippines and India and Mexico, whose populations are already stereotyped in the West as being hardworking and technically competent people ‘inherently’ fit for manual labor, for being given commands and executing them.”
Hu, 2022, xiv
This system in which money is made from “propping up the active user at the expense of the passive server” creates what political theorist Cedric Robinson terms racial capitalism. The racial differences of regional or ethnic groups are exaggerated in order to justify employing such groups for ‘inferior work’, such as transcription (Rev), ‘digital janitor’ (CrowdFlower), or filtering out graphic, offensive content on social media platforms and language-based models like ChatGBT.
It seems the theme consistent throughout the book is the idea that individuals, whether digitally or physically, do not and cannot exist within a vacuum. Even in a so-called liberal, Utopian system of “the Internet”, where users can construct visual identities of themselves without the limitations of a physical body, values such as race and gender are still taken into account. Hu provides the paradox of tech industrialists attempts at inclusivity, striving for more race and gender-neutral workspaces, with the 2016 controversy in which it became public that Facebook provided an option for advertisers “to exclude ads to Black and Latinx groups.” Facebook’s response was to make its internal advertising preferences that capture race as a ‘multicultural affinity’ visible.
“These responses indicate that the technology industry understands race and gender as identity markers– in other words, data values– that can be chosen or are user preferences. Yet by tagging persons of color with interests in ‘African-American culture,’ ‘Asian culture’, or ‘Latino culture’, algorithms contrast them with the default values of whiteness. The inclusion of these ‘affinities’ only reinforce the power a system of classification exerts over those perceived as different: as the poet Edouard Glisant puts it, “I understand your difference… I admit you to existence, within my system.”
Hu, 2022
In Digitizing Race: Visual Cultures of the Internet, published in 2008, Lisa Nakamura explores the concepts of race and gender existing in cyberspace, not just as data, but as artifacts of visual culture and modes of communication between communities with ‘low social power’, such as women and people of color.
Even though these two books are published at least a decade apart, there are still common themes when addressing digital culture. She touches upon Omi and Winant’s theory of racial formation, in which she deposits her theory of digital racial formation, “which would parse the ways that digital modes of cultural production and reception are complicit with this ongoing process”, and concepts of visual capitalism, coined by Lisa Parks in Satellite and Cyber Visualities: Analyzing The Digital Earth Project, as “a system of social differentiation based on users’/viewers’ relative access to technologies of global media.” Nakamura wishes to describe access to the Internet and other technologies not as ‘binary’, but instead, as a spectrum.
“The problematic that I wish to delineate here has to do with parsing the multiple gradations and degrees of access to digital media, and the ways that these shadings are contingent on variables such as class position, race, nationality, and gender.”
Nakamura, 2008
Both authors refer to visual media to break down the response to digital and visual capitalism we currently exist in, and how users operate given the power relations created through binaries such as “user/server”, “spectator/owner”, and “object/representation”. Nakamura provides examples of visual representation on the Internet pre-2008, such as AIM buddy icons, online racial profiling in a Web site called www.alllooksame.com, and online web forums dedicated to providing emotional support to pregnant and conceiving women.

Hu analyzes contemporary and performance art, such as Unfit Bits Metronome (2015) by Tega Brain and Surya Mattu, in which a Fitbit fitness tracking device is strapped to a metronome. He states “For, Brain and Mattu observe, the ‘healthy, active lifestyle is an economic privilege, one that is out of reach (and literally unaffordable) to many working consumers, who may ‘lack sufficient time for exercise or have limited access to sports facilities.”
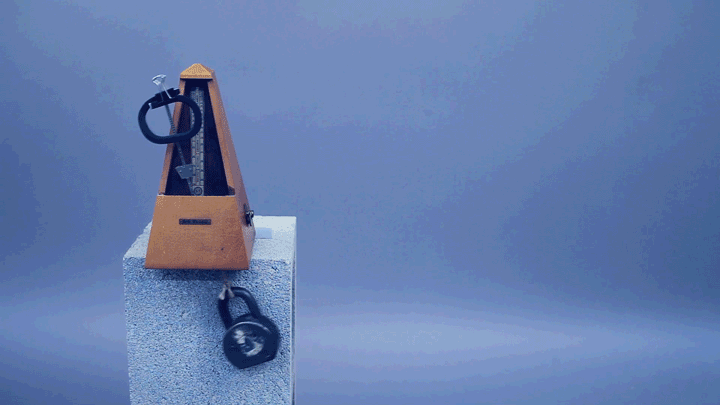
Hu also analyzes the 2011 film Sleeping Beauty directed by Julia Leigh in which he describes the main characters endurance as a passive and unconscious sex-worker to the passivity of users constricted in digital modes of relationality.

The main character, Lucy, takes on a job as a sex worker to make ends meet as a full time student and waitress. The sex work involves her taking a sleeping drug, getting into bed, and sleeping until the evening is over. The subject throughout the film is, however, deadpan and seemingly nihilistic about the situation she finds herself in. In one scene, she is shown lighting a stack of bills on fire right after getting paid.
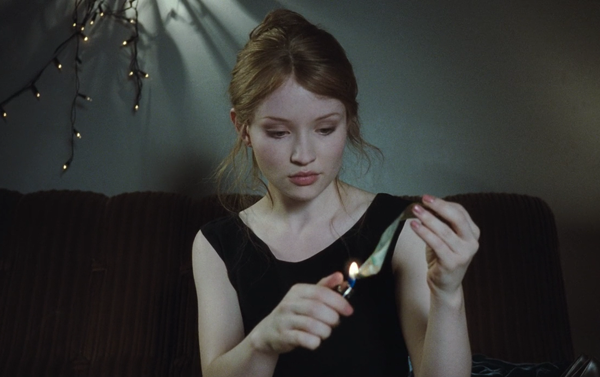
The viewers are challenged at the characters lack of resistance, or even lack of internal struggle displayed on the screen. Hu states “lethargy embraces the potential of being an ‘object being’ as one that relieves a subject of the burden of having to perform aliveness, individuality, and interactivity– all ‘human’ attributes that are actually gendered, ableist, or racially coded.” (Hu, 2022, 120)
Nakamura states in her Introduction:
“So rather than focusing on the idea that women and minorities need to get online, we might ask: How do they use their digital visual capital? In what ways are their gendered and racialized bodies a form of this new type of capital? What sort of laws does this currency operate under? It doesn’t change everything, but what does it change? This brings us back to the privilege of interactivity and its traditional linkage with the creation of a newly empowered subject.”
Hu states in his Introduction:
“For a server, lethargy is the exhaustion of having only a partial claim on selfhood: of needing to ‘be yourself’ for other people, or alternately of having to suppress it; of being what feminist scholars Neda Atanasoski and Kalindi Vora call “human surrogates,” rather than full humans. And yet this is the same problem that afflicts users: a feeling of selfhood as something out-of-reach, burdensome, or even unwanted that trails the feeling of sovereignty like a leaden shadow.”
The books read were interesting, contrasting digital data with digital culture and the implications that follow weaving through concepts of gender and race. As gender has consistently been established as a performative aspect of ones identity, one can question how the performative aspect of gender is translated in visual medium in contrast with data– how do you define data that is gendered on a spectrum of male and female, when the performative aspects are tied to a culture that is every-changing? While one can argue that gender ‘doesn’t matter’, it becomes apparent that even on digital platforms where one can afford to be an anonymous, participating presence in a community, the lived experience of that individual, in reality, is affected by such physical attributes of race and gender, and it is a reality that cannot be ignored.