In Search of Zora/When Metadata Isn’t Enough: Rescuing the Experiences of Black Women Through Statistical Modeling
The authors searched for Alice Walker, who searched for Zora Neale Hurston, in an article that encompasses a generation of searching for cultural and gender visibility in the academic realm related to African American women’s authorship. The research method is based on both quantitative and qualitative analyses of 800,000 documents from the HathiTrust and JSTOR databases. Full article available here.
The research is based on Standpoint Theory, a critical conceptual framework intended to uncover knowledge for both reproducing and dismantling social inequality (Collins, 1990, 1998). This approach encompasses the search, recognition, rescue, and recovery (SeRRR), focusing on utilizing digital technologies to engage with intersectional identities. The authors establish three goals: identifying themes related to African American women using topic modeling, using the identified themes to recover unmarked documents, and visualizing the history of the recovery process.
They argue that social science would greatly benefit from digital technologies, such as big data, speech recognition, and other initiatives like The Orlando Project (2018), the Women Writers Project (Wernimont, 2013, p. 18), and the Schomburg Center’s digital library collection of African American Women Writers of the 19th Century (The New York Library, 1999). These projects challenge dominant power narratives by shedding light on new perspectives and dynamics of minority groups whose history has been overshadowed by the triumphalism of American capitalism, which presents an incomplete view of history from various perspectives.
They emphasized the importance of their quantitative approach over rich and descriptive qualitative research, aiming to create a repository of work that brings more visibility to a different side of the story, which is often uncovered through traditional approaches. They summarized their method in the image below.
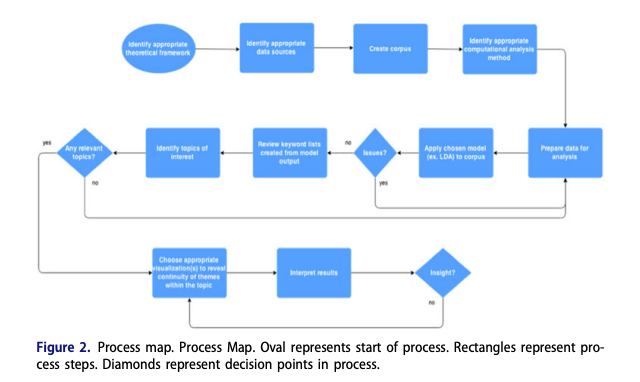
The data collection used the string line (black OR “african american” OR negro) AND (wom?n OR female OR girl) within 800,000 documents from the HathiTrust and JSTOR databases spanning the years 1746 to 2014.
In terms of the analysis method, the authors employed a statistical topic modeling approach to identify documents discussing the experiences of Black women. They utilized a Latent Dirichlet Allocation (LDA) topic modeling technique (Blei, 2012), which clusters words based on the probability of their occurrence across documents using Bayesian probability. Each cluster is referred to as a topic.
They applied the SeRRR method to structure the search and the analysis. The first step is Search and Recognition of the results that are of interest to the Black woman content. In this step, they found 19,398 documents “authored by African Americans based on the metadata with known African American author names and/or phrases such as “African American” or “Negro” within subject headings”: 6,120 of these documents were within the HathiTrust database and 13,278 were within the JSTOR database.
In the second step, the Rescue, they perform a topic analysis in the 19,398 documents. They ended up with 89 initial topics that were reviewed by 5 team members individually – as described in the table below. They used the method of “distant reading”, which can be interpreted as skimming the documents, but focusing on the titles.
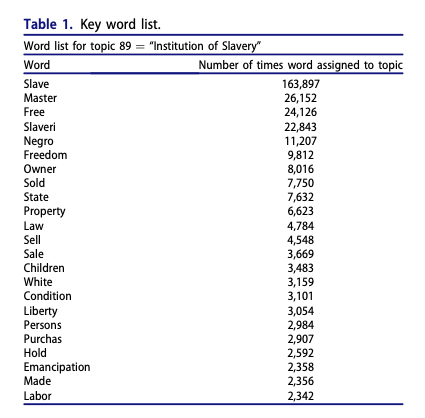
They also developed a method called “intermediate reading” to reflect their reading process that ranges in between the traditional qualitative rich methods and the distant reading of topic modeling. The ultimate goal of this method is to validate the topics and support the quality of the results. The closed this step with a close reading of some full documents for several of these titles.
The third step is Recovery. They successfully rescued and recovered 150 documents previously unidentified related to Black women, including a poem written in 1920 called Race Pride by an African American woman named Lether Isbell.
In conclusion, this article successfully not only applied a research method, but they also contributed to enriching the research repo metadata and making new documents accessible to the academic community.
References
Nicole M. Brown, Ruby Mendenhall, Michael Black, Mark Van Moer, Karen Flynn, Malaika McKee, Assata Zerai, Ismini Lourentzou & ChengXiang Zhai (2019): In Search of Zora/When Metadata Isn’t Enough: Rescuing the Experiences of Black Women Through Statistical Modeling, Journal of Library Metadata, DOI: 10.1080/19386389.2019.1652967 To link to this article: https://doi.org/10.1080/19386389.2019.1652967
Note: this blog post about “How they did it is quite interesting” – here.